XGBoost is an open-source machine learning library that implements optimized gradient boosting algorithms. It is designed to be highly efficient, flexible, and portable, providing parallel tree boosting to solve many data science problems quickly and accurately.
Top 5*
Machine Learning Frameworks
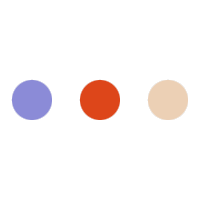
About XGBoost
XGBoost was created in 2014 by Tianqi Chen as part of the Distributed (Deep) Machine Learning Community (DMLC) project. It was developed to improve the speed and performance of gradient boosting algorithms, addressing the need for a more efficient and scalable solution in machine learning competitions and real-world applications.
Strengths of XGBoost include high performance, scalability, and flexibility. It handles missing data well and provides extensive customization options. Weaknesses include complexity in tuning hyperparameters and potential overfitting if not carefully managed. Competitors include LightGBM, CatBoost, and Scikit-learn's Gradient Boosting.
Hire XGBoost Experts
Work with Howdy to gain access to the top 1% of LatAM Talent.
Share your Needs
Talk requirements with a Howdy Expert.
Choose Talent
We'll provide a list of the best candidates.
Recruit Risk Free
No hidden fees, no upfront costs, start working within 24 hrs.
How to hire a XGBoost expert
An XGBoost expert must have strong skills in Python or R programming, proficiency in data preprocessing and feature engineering, and a deep understanding of gradient boosting algorithms. They should also be adept at hyperparameter tuning, model evaluation techniques, and using libraries like NumPy and Pandas for data manipulation.

Matheus H.
Skills
Data scientist with over two years of experience and a degree in Aeronautical Engineering, specializing in data engineering and data science projects. Expertise includes utilizing statistical analysis and machine learning methodologies to solve complex problems, with proficiency in tools such as Python, R, Power BI, and SQL. Recent accomplishments involve developing machine learning models for industrial applications, creating ETL tools for carbon emission reporting, and designing management dashboards to improve decision-making processes. Holds multiple certifications in Data Science and relevant technologies, demonstrating a commitment to continuous professional development.

Rodolfo J.
Skills
Data scientist with over six years of experience specializing in artificial intelligence, machine learning, and statistical analysis across healthcare and industrial sectors. Demonstrated expertise in predictive modeling, time series forecasting, and causal inference, contributing to enhanced population health management and cost control within healthcare frameworks. Proven track record of developing advanced analytical solutions that yielded an 18% improvement in readmission predictions and a significant reduction in forecast inaccuracies through automated processes. Holds an academic background in Chemical Engineering and Complex Data Mining, complemented by certifications in cutting-edge tools such as Docker and PySpark. Proficient in utilizing machine learning frameworks and statistical methods to derive actionable insights and optimize operational efficiency. Multilingual with strong communication and stakeholder engagement skills, focused on driving innovation and delivering substantial results.
*Estimations are based on information from Glassdoor, salary.com and live Howdy data.
USA
$ 224K
Employer Cost
$ 127K
Employer Cost
$ 97K
Benefits + Taxes + Fees
Salary
The Best of the Best Optimized for Your Budget
Thanks to our Cost Calculator, you can estimate how much you're saving when hiring top LatAm talent with no middlemen or hidden fees.